
Conservation Scientist | Writer | Sustainability Professional
Data Science
I leverage a variety of advanced software tools to analyze and interpret ecological data. Primarily using R programming, I also incorporate ArcGIS for spatial analysis to provide comprehensive insights into biodiversity and environmental dynamics. Below are the key data science techniques I specialize in:
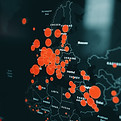
Multivariate Modelling
I specialize in multivariate modeling to analyze complex ecological data and identify relationships between multiple variables. This technique allows me to uncover patterns and interactions in biodiversity and environmental factors, providing a deeper understanding of ecosystems and informing conservation strategies.
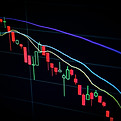
Mixed
Modelling
I have extensive experience in linear mixed and mixed-effects modeling. I apply these methods to account for both fixed and random effects in ecological data. This approach is particularly useful in handling nested or hierarchical data structures, offering robust insights into environmental processes and species behavior while minimizing bias in predictions.

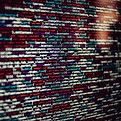
Machine
Learning
Data
Mining
I utilize machine learning techniques, including species distribution modeling, to analyze large datasets and make predictions about species behavior, habitat suitability, and changes in distributions under climate change scenarios. By applying machine learning algorithms, I can process complex data and extract actionable insights that aid in conservation planning and climate resilience strategies.
I incorporate data mining techniques to explore large and complex datasets, identifying trends, patterns, and relationships that might not be immediately apparent. By using data mining tools, I can reveal valuable insights that inform decision-making in biodiversity conservation, restoration, and climate resilience planning.